텍스트 분석이란?
[머신러닝] 텍스트 분석
이전 내용 [머신러닝] 군집화 (Clustering)군집화(Clustering) [군집]군집은 비슷한 샘플을 클러스터 또는 비슷한 샘플의 그룹으로 할당하는 작업으로, 데이터 분석, 고객 분류, 추천 시스템, 검색 엔
puppy-foot-it.tistory.com
이전 내용
[머신러닝] 텍스트 분석: 문서 군집화
텍스트 분석이란? [머신러닝] 텍스트 분석이전 내용 [머신러닝] 군집화 (Clustering)군집화(Clustering) [군집]군집은 비슷한 샘플을 클러스터 또는 비슷한 샘플의 그룹으로 할당하는 작업으로, 데이
puppy-foot-it.tistory.com
문서 유사도
문서와 문서 간의 유사도 비교는 일반적으로 코사인 유사도(Cosine Similarity)를 사용한다.
- 코사인 유사도: 벡터와 벡터 간의 유사도를 비교할 때 벡터의 크기보다는 벡터의 상호 방향성이 얼마나 유사한지에 기반한 것으로, 두 벡터 사이의 사잇각을 구해서 얼마나 유사한지 수치로 적용한 것.
[코사인 유사도가 문서의 유사도 비교에 가장 많이 사용되는 이유]
- 문서를 피처 벡터화 변환하면 차원이 매우 많은 희소 행렬이 되기 쉬운데, 이러한 희소 행렬 기반에서 문서와 문서 벡터 간의 크기에 기반한 유사도 지표 (예. 유클리드 거리 기반 지표)는 정확도가 떨어지기 쉽다.
- 문서가 매우 긴 경우 단어의 빈도수도 더 많을 것이기 때문에 빈도수에만 기반해서는 공정한 비교를 할 수 없다.
- 벡터 크기에 영향 없음: 코사인 유사도는 두 벡터의 방향(패턴)을 비교하여 유사도를 계산하기 때문에, 문서의 길이(또는 크기) 차이가 결과에 영향을 주지 않는다. 이는 길이가 다른 문서들 간의 비교에 유리하다.
- 효율적인 계산: 코사인 유사도는 유클리디안 거리보다 계산이 간단하고 고차원에서도 빠르게 유사도를 계산할 수 있어, 문서 간 유사도 비교를 효율적으로 처리할 수 있다.
- 문서 내용의 패턴을 잘 반영: 코사인 유사도는 벡터의 각도에 따라 유사도를 측정하므로, 단어의 존재 패턴이 비슷한 문서들을 효과적으로 그룹화하며, 특히 단어가 많이 겹치는 유사한 주제의 문서에서 코사인 유사도는 정확한 비교를 가능하게 한다.
▶ 따라서 코사인 유사도는 다양한 길이와 복잡성을 가진 문서들 간에 일관성 있고 신뢰성 높은 유사도 비교를 제공하여 문서 유사도 측정에서 가장 많이 활용된다.
코사인 유사도 기반으로 문서 유사도 구하기
간단한 문서에 대해서 서로 간의 문서 유사도를 코사인 유사도 기반으로 구해 본다.
먼저 두 개의 넘파이 배열에 대한 코사인 유사도를 구하는 cos_simliarity() 함수를 생성한다.
# 문서 유사도
import numpy as np
def cos_similarity(v1, v2):
dot_product = np.dot(v1, v2)
l2_norm = (np.sqrt(sum(np.square(v1))) * np.sqrt(sum(np.square(v2))))
similarity = dot_product / l2_norm
return similarity
doc_list로 정의된 3개의 간단한 문서의 유사도를 비교하기 위해 이 문서를 TF-IDF 로 벡터화된 행렬로 변환한다.
from sklearn.feature_extraction.text import TfidfVectorizer
doc_list = ['if you take the blue pill, the story ends',
'if you take the red pill, you stay in Wonderland',
'if you take the red pill, I show you how deep the rabbit hole goes']
tfidf_vect_simple = TfidfVectorizer()
feature_vect_simple = tfidf_vect_simple.fit_transform(doc_list)
print(feature_vect_simple.shape)
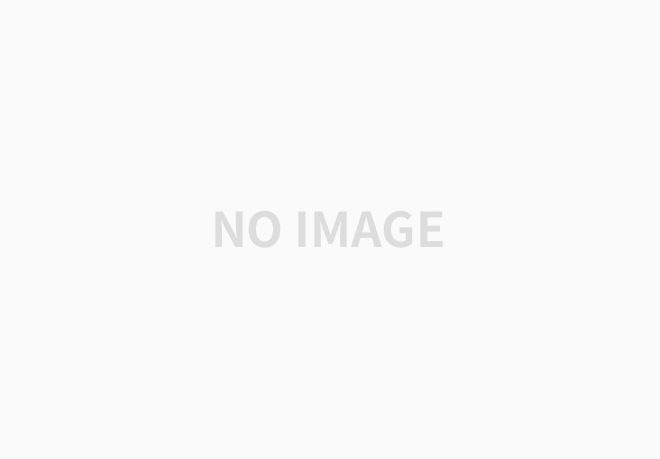
[함수를 이용해 두 개 문서의 유사도 측정]
변환된 행렬은 희소 행렬이므로 앞에서 작성한 cos_similarity() 함수의 인자인 array로 만들기 위해 밀집 행렬로 변환한 뒤 다시 각각을 배열로 변환한다.
- feature_vect_dense[0]은 doc_list 첫 번째 문서의 피처 벡터화이며,
- feature_vect_dense[1]은 doc_list 두 번째 문서의 피처 벡터화이다.
# TfidfVectorizer로 transform()한 결과는 희소 행렬이므로 밀집 행렬로 변환
feature_vect_dense = feature_vect_simple.todense()
# 첫 번째 문장과 두 번째 문장의 피처 벡터 추출
vect1 = np.array(feature_vect_dense[0]).reshape(-1, )
vect2 = np.array(feature_vect_dense[1]).reshape(-1, )
# 첫 번째 문장과 두 번째 문장의 피처 벡터로 두 개 문장의 코사인 유사도 추출
similarity_simple = cos_similarity(vect1, vect2)
print('문장 1, 문장2 cosine 유사도: {0:.3f}'.format(similarity_simple))
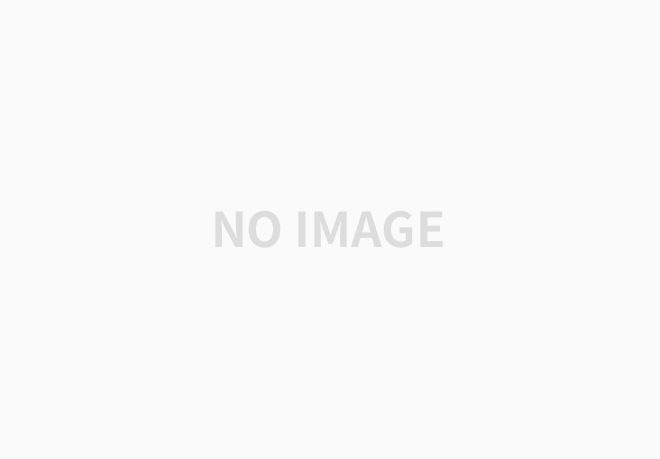
첫 번째 문장과 세 번재 문장, 두 번째 문장과 세 번째 문장의 유사도도 측정해 본다.
vect1 = np.array(feature_vect_dense[0]).reshape(-1, )
vect3 = np.array(feature_vect_dense[2]).reshape(-1, )
similarity_simple = cos_similarity(vect1, vect3)
print('문장 1, 문장3 cosine 유사도: {0:.3f}'.format(similarity_simple))
vect2 = np.array(feature_vect_dense[1]).reshape(-1, )
vect3 = np.array(feature_vect_dense[2]).reshape(-1, )
similarity_simple = cos_similarity(vect2, vect3)
print('문장 2, 문장3 cosine 유사도: {0:.3f}'.format(similarity_simple))
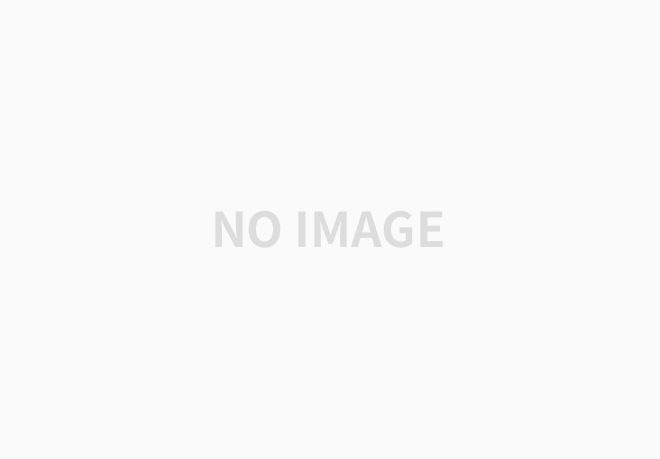
[사이킷런의 API를 이용한 유사도 측정]
사이킷런은 코사인 유사도를 측정하기 위해 sklearn.metrics.pairwise.cosine_similarity API를 제공한다.
cosine_similarity() 함수는 두 개의 입력 파라미터를 받는다.
- 첫 번째 파라미터: 비교 기준이 되는 문서의 피처 행렬
- 두 번째 파라미터: 비교되는 문서의 피처 행렬
cosine_similarity()는 희소 행렬, 밀집 행렬 모두가 가능하며, 행렬 또는 배열 모두 가능하다.
따라서 별도의 변환 작업이 필요 없다.
첫 번째 문서와 비교해 자신 문서인 첫 번째 문서, 그리고 두 번째, 세 번째 문서의 유사도를 측정해본다.
from sklearn.metrics.pairwise import cosine_similarity
similarity_simple_pair = cosine_similarity(feature_vect_simple[0], feature_vect_simple)
print(similarity_simple_pair)
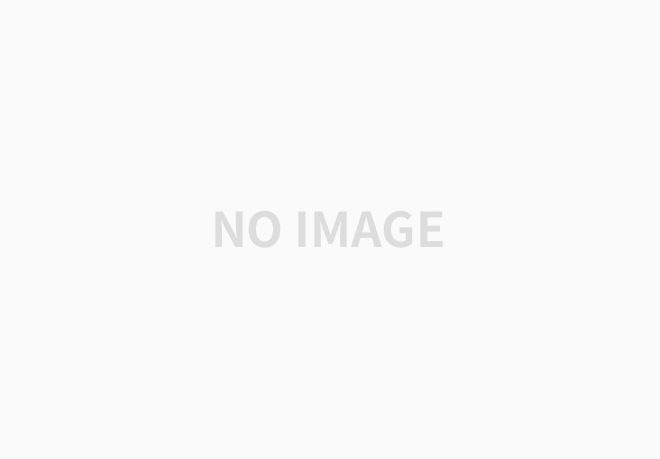
▶ 각 값 별로 첫 번째 문서와 첫 번째 문서와의 유사도 / 첫 번째 - 두 번째 / 첫 번째 - 세 번째 값이다.
만약 자신 문서와의 비교(값 1)를 할 필요가 없다면 비교 대상에서 비교 기준 문서를 제외하면 된다.
from sklearn.metrics.pairwise import cosine_similarity
similarity_simple_pair = cosine_similarity(feature_vect_simple[0], feature_vect_simple[1:])
print(similarity_simple_pair)
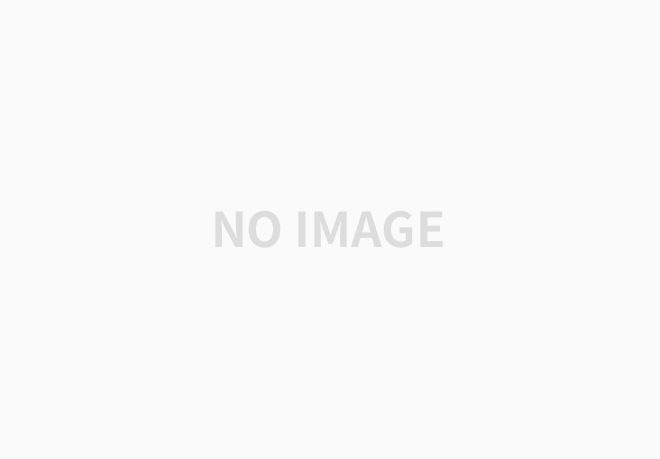
[모든 개별 문서에 쌍으로 코사인 유사도 값 계산]
cosine_similarity() 는 쌍(pair)으로 코사인 유사도 값을 제공할 수 있다.
즉, 1번 - 2, 3번 / 2번 - 1, 3번 / 3번 - 1, 2번 문서의 코사인 유사도를 ndarray 형태로 제공한다.
similarity_simple_pair = cosine_similarity(feature_vect_simple, feature_vect_simple)
print('shape:', similarity_simple_pair.shape)
print(similarity_simple_pair)
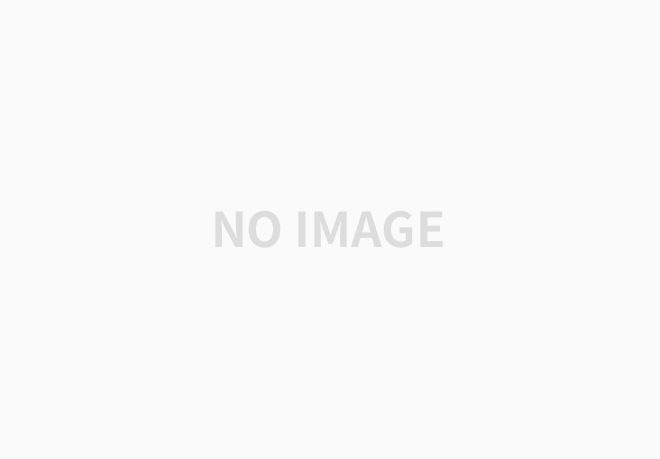
▶ 1행(1열)은 1번 문서 / 2행(2열)은 2번 문서 / 3행(3열)은 3번 문서이다.
Opinion Review 데이터 세트를 이용한 문서 유사도 측정
문서 군집화에서 사용한 Opinion Review 데이터 세트를 이용해 이들 문서 간의 유사도를 측정해 본다.
UCI Machine Learning Repository
This dataset is licensed under a Creative Commons Attribution 4.0 International (CC BY 4.0) license. This allows for the sharing and adaptation of the datasets for any purpose, provided that the appropriate credit is given.
archive.ics.uci.edu
※ 데이터 세트 다운로드 방법은 이전 글(문서 군집화)을 참고하면 된다.
또한, 이전에 생성한 LemNormalize() 함수도 불러와야 한다.
from nltk.stem import WordNetLemmatizer
import nltk
import string
# ord는 문자의 유니코드 값을 반환, string.punctuation은 구두점
remove_punct_dict = dict((ord(punct), None) for punct in string.punctuation)
lemmar = WordNetLemmatizer()
def LemTokens(tokens) :
return [lemmar.lemmatize(token) for token in tokens]
def LemNormalize(text) :
return LemTokens(nltk.word_tokenize(text.lower().translate(remove_punct_dict)))
import pandas as pd
import glob, os
from sklearn.feature_extraction.text import TfidfVectorizer
from sklearn.cluster import KMeans
import warnings
warnings.filterwarnings('ignore')
pd.set_option('display.max_colwidth', 700)
path = r"C:/Users/niceq/Documents/DataScience/Python ML Guide/Data/OpinosisDataset1.0/topics"
# path로 지정한 디렉터리 밑에 있는 모든 .data 파일들의 파일명을 리스트로 취합
all_files = glob.glob(os.path.join(path, "*.data"))
filename_list = []
opinion_text = []
# 개별 파일들의 파일명은 filename_list 로 취합
# 개별 파일들의 파일 내용은 DataFrame 로딩 후 다시 String으로 변환하여 opnion_text 리스트로 취합
for file_ in all_files:
# 개별 파일을 읽어서 DataFrame으로 생성
df = pd.read_table(file_, index_col=None, header=0, encoding='latin1')
#절대 경로로 주어진 파일명 가공. 확장자(.data) 제거
filename_ = file_.split('\\')[-1]
filename = filename_.split('.')[0]
# 파일명 리스트와 파일 내용 리스트에 파일명과 파일 내용 추가
filename_list.append(filename)
opinion_text.append(df.to_string())
# 파일명 리스트와 파일 내용 리스트를 DataFrame으로 생성
document_df = pd.DataFrame({'filename':filename_list, 'opinion_text':opinion_text})
tfidf_vect = TfidfVectorizer(tokenizer=LemNormalize, stop_words='english',
ngram_range=(1,2), min_df=0.05, max_df=0.85)
# opinion_text 칼럼값으로 feature vectorization 수행
feature_vect = tfidf_vect.fit_transform(document_df['opinion_text'])
km_cluster = KMeans(n_clusters=3, max_iter=10000, random_state=0)
km_cluster.fit(feature_vect)
cluster_label = km_cluster.labels_
cluster_centers = km_cluster.cluster_centers_
document_df['cluster_label'] = cluster_label
document_df.head()
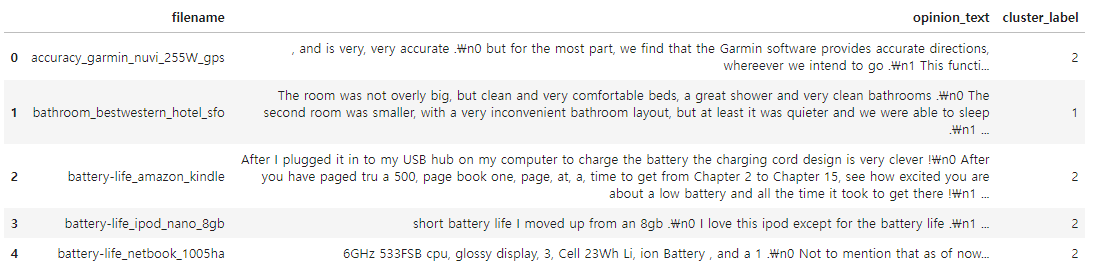
▶ 해당 문서의 군집화는 전자제품, 호텔, 자동차를 주제로 군집화되었다.
[특정 문서와 다른 문서 간의 유사도 측정]
이 중 호텔을 주제로 군집화된 문서를 이용해 특정 문서와 다른 문서 간의 유사도를 알아본다.
문서를 피처 벡터화해 변환하면 문서 내 단어(Word)에 출현 빈도와 같은 값을 부여해 각 문서가 단어 피처의 값으로 벡터화된다. 이렇게 각 문서가 피처 벡터화된 데이터를 cosine_similarity()를 이용해 상호 비교하여 유사도를 확인한다.
먼저 호텔을 주제로 군집화된 데이터를 먼저 추출하고 이 데이터에 해당하는 TfidfVectorizer의 데이터를 추출하는데, 별도의 벡터화를 수행하지 않고, TfidfVectorizer로 만들어진 데이터에서 그대로 추출한다.
- DataFrame 객체 변수인 document_df 에서 먼저 호텔로 군집화된 문서의 인덱스 추출
- 추출된 인덱스를 그대로 이용해 TfidfVectorizer 객체 변수인 feature_vect에서 호텔로 군집화된 문서의 피처 벡터 추출
그전에, 호텔을 주제로 군집화된 데이터의 label 이 몇 번인지 확인해야 한다.
# (군집이 3개 이므로)숫자에 0~2 사이의 숫자 입력
document_df[document_df['cluster_label']==숫자].sort_values(by='filename')
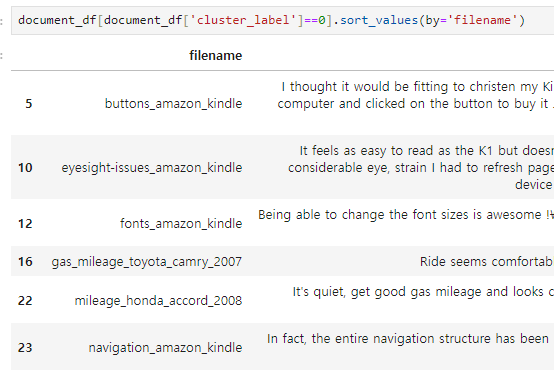
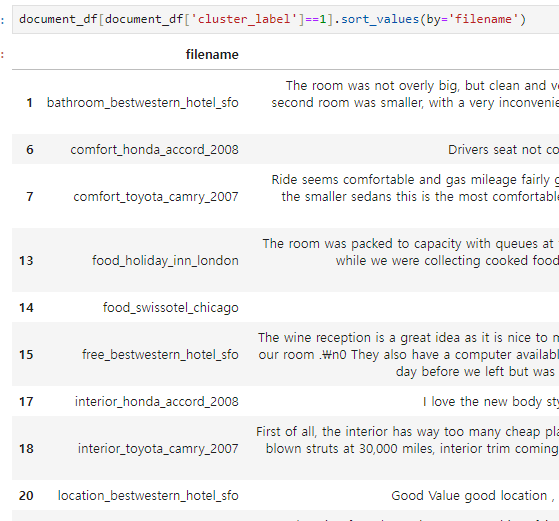
▶ 조회 결과, 1번 label이 호텔로 군집화된 데이터임을 알 수 있다.
from sklearn.metrics.pairwise import cosine_similarity
# cluster_label=1인 데이터는 호텔로 군집화된 데이터임. DataFrame에서 해당 인덱스를 추출
hotel_indexes = document_df[document_df['cluster_label']==1].index
print('호텔로 클러스터링 된 문서들의 DataFrame Index:', hotel_indexes)
# 호텔로 군집화된 데이터 중 첫 번째 문서를 추출해 파일명 표시
comparison_docname = document_df.iloc[hotel_indexes[0]]['filename']
print('#### 비교 기준 문서명 ', comparison_docname, '와 타 문서 유사도 ####')
'''document_df에서 추출한 Index 객체를 feature_vect로 입력해 호텔 군집화된 feature_vect 추출
이를 이용해 호텔로 군집화된 문서 중 첫 번째 문서와 다른 문서 간의 코사인 유사도 측정.'''
simliarity_pair = cosine_similarity(feature_vect[hotel_indexes[0]], feature_vect[hotel_indexes])
print(simliarity_pair)

[유사도 정렬 및 시각화]
단순히 숫자로만 표시해서는 직관적으로 문서가 어느 정도 유사도를 가지는지 이해하기 어려울 수 있으므로 유사도가 높은 순으로 정렬하고 시각화한다.
cosine_similarity()는 쌍 형태의 ndarray를 반환하므로 이를 판다스 인덱스로 이용하기 위해 reshape(-1)로 차원을 변경한다.
import seaborn as sns
import numpy as np
import matplotlib.pyplot as plt
%matplotlib inline
simliarity_pair = cosine_similarity(feature_vect[hotel_indexes[0]], feature_vect[hotel_indexes])
# 첫 번째 문서와 타 문서간 유사도가 큰 순으로 정렬한 인덱스 추출하되, 자기 자신은 제외
sorted_index = simliarity_pair.argsort()[:, ::-1]
sorted_index = sorted_index[:, 1:]
# 유사도가 큰 순으로 hotel_indexes를 추출하여 재정렬
hotel_sorted_indexes = hotel_indexes[sorted_index.reshape(-1)]
# 유사도가 큰 순으로 재정렬(자기 자신 제외)
hotel_1_sim_value = np.sort(simliarity_pair.reshape(-1))[::-1]
hotel_1_sim_value = hotel_1_sim_value[1:]
# 유사도가 큰 순으로 정렬된 인덱스와 유사도 값을 이용해 파일명과 유사도 값을 막대 그래프로 시각화
hotel_1_sim_df = pd.DataFrame()
hotel_1_sim_df['filename'] = document_df.iloc[hotel_sorted_indexes]['filename']
hotel_1_sim_df['similarity'] = hotel_1_sim_value
print('가장 유사도가 큰 파일명 및 유사도:\n', hotel_1_sim_df.iloc[0, :])
sns.barplot(x='similarity', y='filename', data=hotel_1_sim_df)
plt.title(comparison_docname)
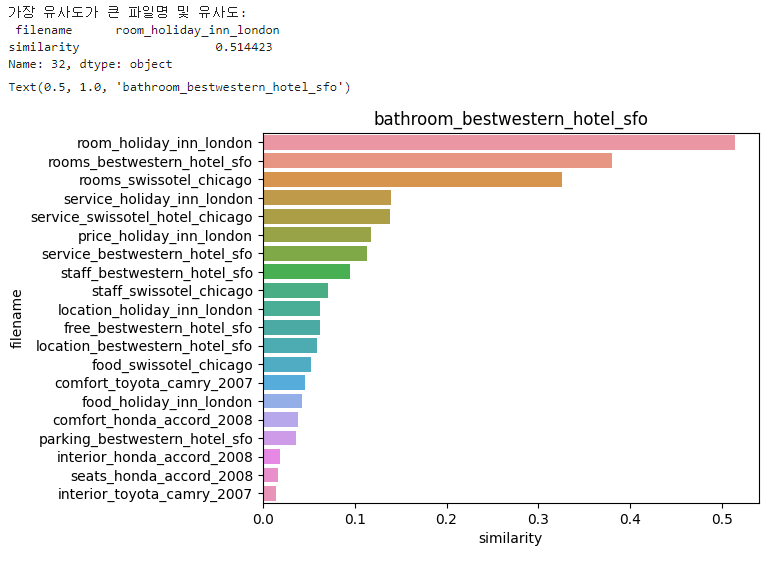
▶ 첫 번째 문서인 베스트 웨스턴 호텔 화장실 리뷰(bathroom_bestwestern_hotel_sfo)와 가장 비슷한 문서는 'room_holiday_inn_london'이며, 약 0.5144의 코사인 유사도 값을 나타내고 있다.
[전자 제품을 주제로 군집화된 문서의 유사도 및 시각화]
앞서, cluster_label 조회 시, 2번 label은 전자 제품 리뷰로 군집화된 문서임을 파악할 수 있었다.
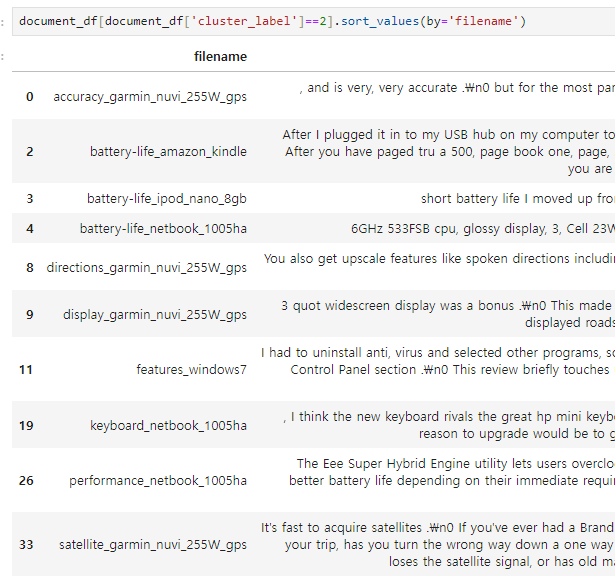
위의 호텔 문서 군집화 유사도 측정 및 시각화 코드를 응용하여 전자 제품 문서 역시 동일한 작업을 수행해 본다.
# cluster_label=1인 데이터는 호텔로 군집화된 데이터임. DataFrame에서 해당 인덱스를 추출
elec_indexes = document_df[document_df['cluster_label']==2].index
print('전자 제품으로 클러스터링 된 문서들의 DataFrame Index:', elec_indexes)
# 전자 제품으로 군집화된 데이터 중 첫 번째 문서를 추출해 파일명 표시
comparison_docname_1 = document_df.iloc[elec_indexes[0]]['filename']
print('#### 비교 기준 문서명 ', comparison_docname_1, '와 타 문서 유사도 ####')
simliarity_pair_1 = cosine_similarity(feature_vect[elec_indexes[0]], feature_vect[elec_indexes])
print(simliarity_pair_1)
# 첫 번째 문서와 타 문서간 유사도가 큰 순으로 정렬한 인덱스 추출하되, 자기 자신은 제외
sorted_index_1 = simliarity_pair_1.argsort()[:, ::-1]
sorted_index_1 = sorted_index_1[:, 1:]
# 유사도가 큰 순으로 elec_indexes를 추출하여 재정렬
elec_sorted_indexes = elec_indexes[sorted_index_1.reshape(-1)]
# 유사도가 큰 순으로 재정렬(자기 자신 제외)
elec_1_sim_value = np.sort(simliarity_pair_1.reshape(-1))[::-1]
elec_1_sim_value = elec_1_sim_value[1:]
# 유사도가 큰 순으로 정렬된 인덱스와 유사도 값을 이용해 파일명과 유사도 값을 막대 그래프로 시각화
elec_1_sim_df = pd.DataFrame()
elec_1_sim_df['filename'] = document_df.iloc[elec_sorted_indexes]['filename']
elec_1_sim_df['similarity'] = elec_1_sim_value
print('가장 유사도가 큰 파일명 및 유사도:\n', elec_1_sim_df.iloc[0, :])
sns.barplot(x='similarity', y='filename', data=elec_1_sim_df)
plt.title(comparison_docname_1)
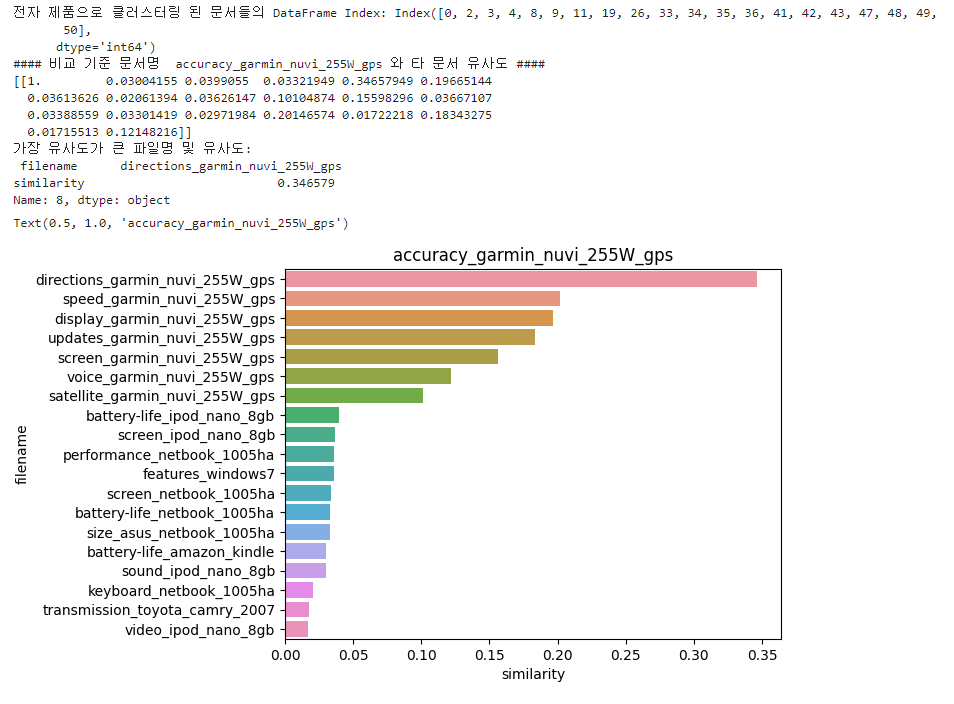
▶ 첫 번째 문서인 'accuracy_garmin_nuvi_255W_gps (아마 네비게이션인듯 하다)'와 가장 비슷한 문서는 'directions_garmin_nuvi_255W_gps' 이며, 약 0.346의 유사도 값을 나타내고 있다.
다음 내용
[머신러닝] 텍스트 분석: 한글 텍스트 처리
텍스트 분석이란? [머신러닝] 텍스트 분석이전 내용 [머신러닝] 군집화 (Clustering)군집화(Clustering) [군집]군집은 비슷한 샘플을 클러스터 또는 비슷한 샘플의 그룹으로 할당하는 작업으로, 데이
puppy-foot-it.tistory.com
[출처]
파이썬 머신러닝 완벽 가이드
wikidocs.net - 05-01 코사인 유사도(Cosine Similarity) - 딥 러닝을 이용한 ...
velog.io - [NLP] 문서 유사도 및 언어 모델
tistory.com - [딥러닝을 이용한 자연어 처리 입문] 5. 벡터의 유사도(Vector ...
velog.io - 문서 유사도 검사ㅣBoW, TF-IDF, Word2Vec, FastText, ...
naver.com - 문서분류 - cosine 유사도 사용 이유 - 네이버 블로그
'[파이썬 Projects] > <파이썬 머신러닝>' 카테고리의 다른 글
[머신러닝] 텍스트 분석 실습 - 캐글 Mercari (1) | 2024.11.01 |
---|---|
[머신러닝] 텍스트 분석: 한글 텍스트 처리 (6) | 2024.10.31 |
[머신러닝] 텍스트 분석: 문서 군집화 (2) | 2024.10.31 |
[머신러닝] 텍스트 분석: 토픽 모델링 (4) | 2024.10.31 |
[머신러닝] 텍스트 분석: 감성 분석 (3) | 2024.10.30 |